

Return 255 - cv2.filter2D(src=inverted, ddepth=-1, kernel=self.kernel)ĭef _call_(self, frame: np.ndarray) -> np.ndarray:įrame: (np.ndarray) - frame to excecute pencil sketch onįrame: (np.ndarray) - processed frame that is pencil sketch type
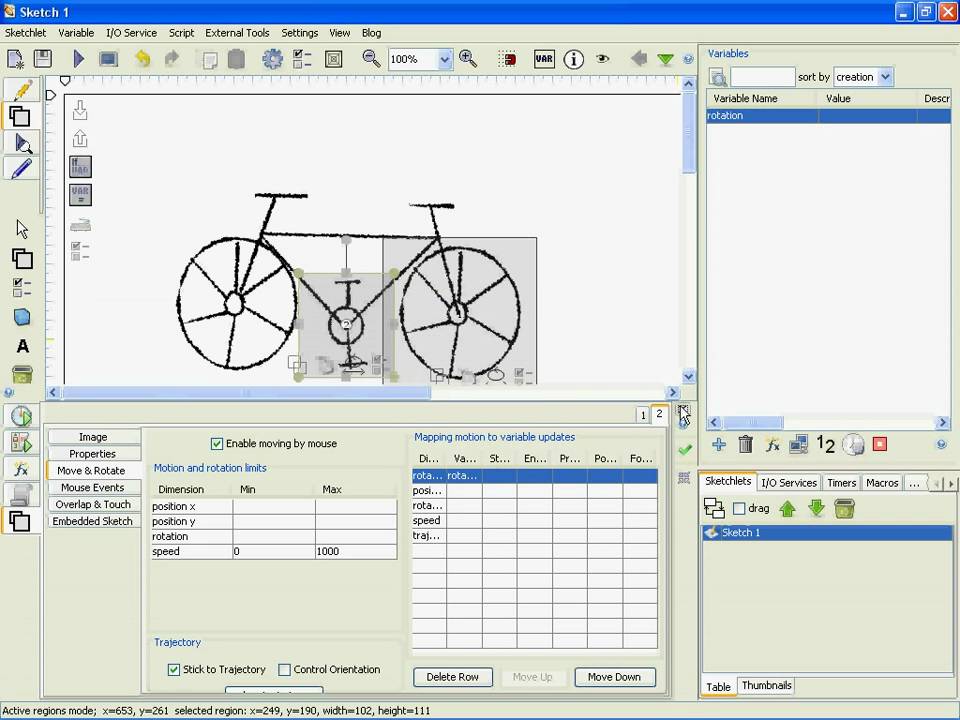
If self.sharpen_value is not None and isinstance(self.sharpen_value, int): Image: (np.ndarray) - image to be sharpened Self.kernel = np.array(,, ]) if kernel = None else kernelĭef dodge(self, front: np.ndarray, back: np.ndarray) -> np.ndarray:ĭef sharpen(self, image: np.ndarray) -> np.ndarray: Kernel: (np.ndarray) - custom kernel to apply in sharpen function Sharpen_value: (int) - sharpen value to apply in predefined kernel array Ksize: (float) - ratio to apply for cv2.GaussianBlur """Apply pencil sketch effect to an imageīlur_simga: (int) - sigma ratio to apply for cv2.GaussianBlur
#Sketchify a picture code
Here is the complete pencil sketch code in the object: class PencilSketch: def dodge(self, front: np.ndarray, back: np.ndarray) -> np.ndarray:įront: (np.ndarray) - front image to be applied to dodge algorithmīack: (np.ndarray) - back image to be applied to dodge algorithmįinal_img = self.dodge(blur_img, grayscale) We have a blurry image that highlights the boldest edges. This brightens the lower layer depending on the value of the upper layer. The results of the blurred image look following:Ĭolour Dodge blending mode splits the bottom layer from the inverted top layer.
#Sketchify a picture trial
A suitable value of sigma can be chosen by trial and error: blur_img = cv2.GaussianBlur(inverted_img, ksize=(0, 0), sigmaX=5 Sigma controls the amount of dispersion and, therefore, the degree of blurring. As sigma increases, the picture becomes blurrier. The most important thing here is the variance of the Gaussian function or sigma. Blurring is performed by applying a Gaussian filter to the inverted image. When we display the inverted image or save it on a disc, we receive the following picture: Because, by default, images are 8bit and have a maximum of 256 tones: inverted_img = 255 - grayscale It's as simple as simply subtracting 255 from each image pixel. When I am telling invert, I mean white should become black and wise versa. Because of that, we need to return back to 3 layers image we do it with the numpy stack function.

Here we are multiplying RGB image channels with appropriate values and concatenating them to a single channel. Grayscale = np.stack((grayscale,) * 3, axis=-1) But not going into the math, the formula will look following: grayscale = np.array(np.dot(frame, ), dtype=np.uint8) But we can easily convert our image to grayscale, knowing the math behind that. But numpy doesn't have any built-in function for grayscaling. We can do either with the cv2 library or numpy. When these lines are executed, the image will open in a new window with a title as 'image':įirst, what we need to do with our image, is to grayscale it (convert it to black and white). The following OpenCV commands can be used to display the image on the screen: cv2.imshow('image', frame)
#Sketchify a picture how to
The next important step while creating such a sketch in our project is to know how to quickly view the results without saving them on disc. But as I mentioned, this can be a sequence of frames or an image loaded by other methods. This command reads the file " image.png" located in the current folder on the disc and stored in memory as a frame. Here is one of the commands that can be used to read an image stored on a disc using OpenCV: frame = cv2.imread("porche.png") We import them with two following lines of code: import cv2 OpenCV and Numpy are the only libraries that are needed for the project. I'll do this to expand the functionality of the background-removal project that I am working on in this tutorial series. But I'll demonstrate how to create an object we can apply to any image, video, or real-time stream. Apply the Dodge blend to the blurred and grayscale image.In this tutorial, I'll show you how we can create a "pencil" sketch image with just a few lines of code. So, in the previous tutorials, we learned how to separate ourselves from the background, detect faces, and do all of this in real-time. So we can do various matrix manipulations to get exciting results. A picture is an array of numbers in Python. I've always been fascinated by computer vision, especially its power to manipulate images in rapid matrix multiplication.
